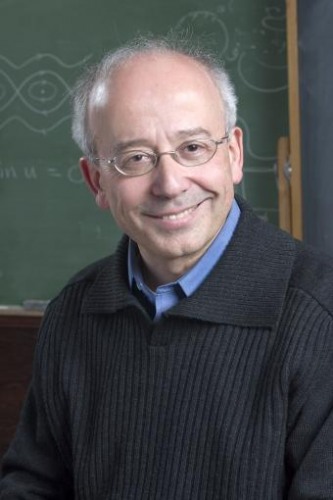
Elie Bienenstock
Office Hours: by appointment
Biography
Ph.D., Brown University, 1980
Elie Bienenstock studies the mechanisms used by brains to create and compose complex representations. His research, focusing on models of vision, assumes that brains use compositional hierarchies of explicit and detailed representations of objects, parts, and relationships. With colleagues in neuroscience and applied math, he investigates the hypothesis that the fine temporal structure of cortical activity, e.g. the synchronous firing of neurons, plays an important role in these representations.
Biography
The first part of my research is in computer vision - how computers interpret images. I'm trying to contribute to the understanding of object recognition, which is part of artificial intelligence. Among its practical uses are military target recognition and optical character recognition in which an image is transformed into text. The main goal is to interpret highly ambiguous images, a task that is not solved very well by current algorithms. What characterizes our approach is that it is compositional. A face is made of eyes, nose, mouth, and each of these is made of simpler constituents, and there are rules that guide how these constituents come together in an image. We try to derive composition rules by studying natural images. We also try to learn the parameters of these rules by statistical methods. The other part of my research is in theoretical neuroscience. We are searching animal behavior data for specific temporal patterns that may shed light on the mechanisms used by the brain to construe separate parts into a whole object.
I love the interdisciplinary aspect of this research. I was attracted by both the mathematics and the neuroscience in it. I like to use my imagination to create theories that are abstract by their nature but can be tested.
Research Interests
The nature of the code that our brains use to create, store, retrieve, match and compose—and more generally compute with—neural representations of external events, stimuli, sensations or actions still largely eludes us. My research, carried out in collaboration with colleagues from the Departments of Neuroscience, Applied Mathematics and Computer Science, attempts to contribute to the understanding of brain codes, using a number of mathematical/numerical tools. These range from the the study of mathematical models of natural and artificial vision systems to the statistical analysis of large volumes of cortical activity recorded from behaving monkeys, or the analysis or fMRI data recorded from humans engaged in various sensory-motor tasks.
Our models of vision focus on the issues of invariant shape recognition and interpretation of images that are locally ambiguous—as are most images of natural scenes. We believe that the remarkable capacities of our brains at interpreting such images are predicated on the use of compositional hierarchies of explicit and detailed neural representations for objects/actions, their parts, and the various relationships that exist between them. We actively investigate, on both the theoretical and the experimental levels, the hypothesis that these representations are physically couched in the fine temporal structure of cortical activity, in particular in deviations from statistical independence of firing of distinct neurons, manifested by an excess of synchrony measured on the millisecond scale.
Teaching
APMA 0340 (Introduction to Differential Equations, Part II)
APMA 0410 (Mathematical Methods in the Brain Sciences)
APMA 1650 (Statistical Inference, Part I)
APMA 1660 (Statistical Inference, Part II)
APMA 1670 (Statistical Analysis of Time Series)
NEUR 1680 (Computational Neuroscience)
Students
Yun Gao