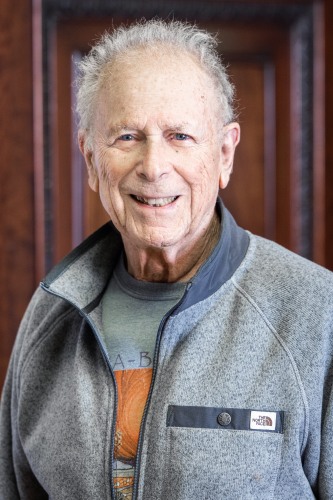
Harold Kushner
Biography
Ph.D., University of Wisconsin, 1958
Professor Kushner's current research interests include stochastic control and stochastic systems theory, approximation methods, limit theorems and optimization methods for complex stochastic systems, and stochastic networks (such as heavy traffic theory and control). Professor Kushner's work also involves nonlinear filtering, applications to adaptive and competitive learning processes and in communication theory.
BIOGRAPHY
Dr. Kushner received the Ph.D. in Electrical Engineering from the University of Wisconsin in 1958. He worked at Lincoln Laboratories and RIAS before coming to Brown in 1964 with the group that formed the Lefschetz Center for Dynamical Systems. He is a former director of that center as well as a former chairman of the Applied Mathematics Department, and is currently a University Professor Emeritus.
Since then, in ten books and well over two hundred papers, he has established a substantial part of modern stochastic systems theory. These include seminal developments of stochastic stability for both Markovian and non-Markovian systems, optimal nonlinear filtering and effective algorithms for approximating optimal nonlinear filters, stochastic variational methods and the stochastic maximum principle, numerical methods for jump-diffusion type control and game problems (the current methods of choice), efficient numerical methods for Markov chain models, methods for singularly perturbed stochastic systems, an extensive evelopment of controlled stochastic networks such as queueing/ communications systems under conditions of heavy traffic, methods for the analysis and approximation of systems driven by wideband noise, large-deviation methods for control problems with small noise effects, stochastic distributed and delay systems, and nearly optimal control and filtering for non-Markovian systems. His work on stochastic approximations and recursive algorithms has set much of the current framework, and he has contributed heavily to applications of control methods to communications problems.
INTERESTS
In the mid-1960s, Dr. Kushner established much of the basic theory of stochastic stability, based on the concept of supermartingales as Lyapunov functions. Extension to non-Markovian and infinite-dimensional systems followed, and he developed a full stochastic analog of the LaSalle invariance theorem, which is essential for application to distributed and delay systems. Such results, whicb are analogous to the theory for deterministic systems, are essential for the analysis of the long-term behavior of complex stochastic systems arising in control, economics, recursive algorithms, and elsewhere. Because proof of stability is often required before any further analysis can be done, these have been fundamental tools for the analysis of stochastic systems ever since. It was Kushner who, in the mid- 1960s, provided the first rigorous development of nonlinear filters for diffusion-type processes with white observation noise. This is the analog of Kalman filtering for nonlinear systems, and concerns the tracking of systems with nonlinear dynamics or observations. He also developed many practical algorithms for approximating optimal filters, adaptations of the theory for dealing with robustness and for systems that are only "approximately" Markovian or have only widebandwidth noise, as well as extensions to distributed systems.
Numerical methods for problems arising in stochastic control have always been a challenge. The optimal value function formally solves the Bellman-Hamilton-Jacobi equation. Even when the derivation is formally justified, the equation can be a highly nonlinear (even in the highest-order terms) elliptic or parabolic partial differential equation, an integral-differential equation, or a variational inequality. The equations tend to be degenerate and to have serious singularities; moreover, the reflections on the boundaries are often not continuous. The solutions, even when they are known to exist, might not be differentiable or even continuous. Generally, there is little theory concerning regularity or even existence. So the use of classical numerical methods can be problematic. Kushner's Markov chain approximation method is the current approach of choice for such problems. The algorithms are robust; they are intuitively reasonable and have physical meaning because the approximating Markov chains represent systems similar to the one being approximated. The convergence theory is purely probabilistic, using methods of stochastic control, so that the analytical difficulties are avoided. Kushner is the author of ten books and more than two hundred papers. In his 1984 book he developed a comprehensive approach to approximation and weak convergence methods for random processes, with emphasis on problems that arise in control and communications, e.g., systems driven by wide-band noise, perhaps appearing nonlinearly in the dynamics. The original physical models are often not Markovian; but for purposes of analysis or numerical approximation, approximation by a Markovian model is important. The book presents powerful methods for obtaining such approximations, as well as for proving stability of the original physical systems. The ideas are widely used in the analysis of stochastic recursive algorithms, for the approximation of stochastic networks under general conditions, and for obtaining "nearly optimal" controls for systems driven by wide-bandwidth noise; recently, they have been applied to the development of scheduling algorithms for mobile communications with rapidly varying connecting channels.
His 1990 book sets out a complete theory of singularly perturbed stochastic control systems and nonlinear filters, with multiple time scales and white or wide-band noise processes. Most recently, his 2001 book presents a thorough development of the theory of heavy traffic analysis of both controlled and uncontrolled queueing and
communications systems.
With his work in stochastic approximation and recursive stochastic algorithms, Kushner put in place a large part of the current framework. The theory concerns the analysis of the asymptotic properties of the paths of a large class of stochastic difference equations. Such equations model a large number of adaptive processes in control and communications, learning in neural networks, market-adjustment algorithms in economics, and processes in other settings. They are ubiquitous in current applications. Kushner established powerful methods for the analysis of convergence and rate of convergence under very weak conditions on the noise and dynamics.